True but it doesn't boost recovery, it just counts repetitions which doesn't help until we get EXACT NUMBER OF REPETITIONS NEEDED PER 100% RECOVERY PROTOCOLS!
Does no one in stroke understand anything about recovering from a stroke?
Primseq Digital Tool Shows Accurate Movement Tracking in Stroke Rehabilitation
The digital tool was used to evaluate more than 12,500 recorded upper body movements among a cohort of 41 adults undergoing poststroke rehabilitation exercises.
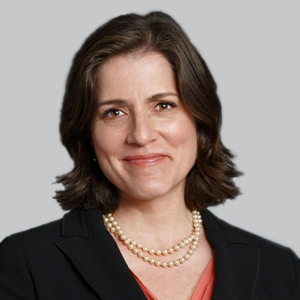
Heidi Schambra, MD
New data published in PLOS Digital Health suggest that a novel digital tool called Primseq, a sensor-equipped computer program, can aid in patients’ recovery from stroke with accurate tracking of the movement intensity during stroke rehabilitation therapy.1,2
The tool showed that it was 77% effective in identifying and counting arm motions prescribed to patients as part of their stroke rehabilitation exercises. Using sensors strapped to the arms and back, the tool assessed 12,545 recorded movements according to their function. The study included 41 adults post stroke who were prescribed rehabilitation movements—using a fork to eat and using a comb in their hair—resulting in more than 51,000 upper body movements recorded from Primseq’s 9 sensors. The artificial intelligence machine learning platform was then used to match the recorded movements to patterns and functional categories.1,2
“Knowing how much physical rehabilitation stroke patients need to recover has been hampered by the inability to easily count training movements,” cosenior investigator Heidi Schambra, MD, associate professor, Department of Neurology and Department of Rehabilitation Medicine, NYU Langone, told NeurologyLive®. “Here, we show that it is possible to identify and count training movements in the impaired arm with an approach that uses wearable motion sensors and machine learning. Our measurement tool is an exciting step toward objectively capturing and dosing rehabilitation to maximize recovery.
READ MORE: NeuroVoices: Michael Sughrue, MD, on Using Machine Learning to Build a Better Brain Map
Schambra and colleagues noted in an NYU news release that they intend to use Primseq to define the intensity of movements in order to select those that promote recovery toward independent movement post stroke. She told NeurologyLive® that the investigators plan to streamline the wearable sensor array and package the algorithm into clinical software to improve the tool’s user-friendliness. She and colleagues intend to make PrimSeq freely available worldwide to stroke rehabilitation professionals and have posted the data used to construct the program online here: SimTK wesbite: Primseq.3
“Ultimately, it will be important to have this tool readily available to researchers and therapists, and to this end, we have made our machine learning algorithm freely available. This tool could be used by researchers to identify training intensities that work best to maximize recovery. It could also be used by therapists to track and adjust training during a rehabilitation session,” Schambra said. “We will continue to refine the tool and improve its measurement performance by training it on additional stroke patients.”
Existing preclinical literature suggests that upper body exercise of certain intensities can promote recovery post stroke, but in-human research has suggested that patients with stroke receive one-tenth of the exercise training proven effective in animals, on average. Part of this challenge has been attributed to the difficulty in tracking movements. Per the US Centers for Disease Control and Prevention almost 800,000 Americans suffer strokes annual, and arm mobility—as well as for other limbs—is seriously reduced in more than 50% of these patients.4
“PrimSeq has state-of-the-art performance in terms of identifying and counting functional movements in stroke patients, and we are gathering more data to continue increasing its accuracy,” cosenior investigator Carlos Fernandez-Granda, PhD, associate professor of mathematics and data science, New York University, said in a statement.1
With regard to the future assessments of such a tool, Schembra and colleagues acknowledged some limitations of the tool, namely that it lacks measurements of how often the exercises/movements are performed, which they wrote, "is important for tracking recovery and tailoring rehabilitation." Additionally, they suggested characterizing and referencing normative kinematics of the primitive varieties to "generate continuous measurements of abnormal primitive performance." As well, they noted that PrimSeq's clinical utility could be boosted with additional model training and real-world refinement. "Finally, the classification performance of Seq2Seq was limited in some cases (e.g. stabilizations, tooth-brushing activity). Future work could employ alternative deep learning models with explainable artificial intelligence to identify sources of confusion, which could then be targeted to improve classification performance," they wrote.
No comments:
Post a Comment