No clue.
Federated Joint Learning of Robot Networks in Stroke Rehabilitation
Abstract
Advanced by rich perception and precise execution, robots possess immense potential to provide professional and customized rehabilitation exercises for patients with mobility impairments caused by strokes. Autonomous robotic rehabilitation significantly reduces human workloads in the long and tedious rehabilitation process. However, training a rehabilitation robot is challenging due to the data scarcity issue. This challenge arises from privacy concerns (e.g., the risk of leaking private disease and identity information of patients) during clinical data access and usage. Data from various patients and hospitals cannot be shared for adequate robot training, further compromising rehabilitation safety and limiting implementation scopes. To address this challenge, this work developed a novel federated joint learning (FJL) method to jointly train robots across hospitals. FJL also adopted a long short-term memory network (LSTM)-Transformer learning mechanism to effectively explore the complex tempo-spatial relations among patient mobility conditions and robotic rehabilitation motions. To validate FJL’s effectiveness in training a robot network, a clinic-simulation combined experiment was designed. Real rehabilitation exercise data from 200 patients with stroke diseases (upper limb hemiplegia, Parkinson’s syndrome, and back pain syndrome) were adopted. Inversely driven by clinical data, 300,000 robotic rehabilitation guidances were simulated. FJL proved to be effective in joint rehabilitation learning, performing 20% - 30% better than baseline methods.
I INTRODUCTION
Stroke is a global healthcare problem contributing to individual disability and death [1]; rehabilitation typically aims to train patients in compensatory strategies with proximal (e.g., shoulder abduction, arm flexion) and distal (e.g., hand open, finger extension) movements to facilitate patient recovery on strength, speed, endurance, and precision of multijoint movements [1, 2]. While training a human expert for professional rehabilitation is expensive and lengthy, for example, an attending physician-level expert will need an average professional education and training time of 8-11 years and 0.2-0.5 million dollars [3].
Powered by sensor and control technologies, robots can precisely and durably provide patient training exercises to ensure quality rehabilitation exercise, significantly reducing human workload and economic/time costs; most importantly, powered by the latest learning algorithms, a robot with expert-level skills can be trained within several days [4]. Therefore, it is promising to rely on robots for durable, reliable, and economical rehabilitation for movement disorder stroke diseases, such as hemorrhagic stroke and hemiplegic stroke [5].
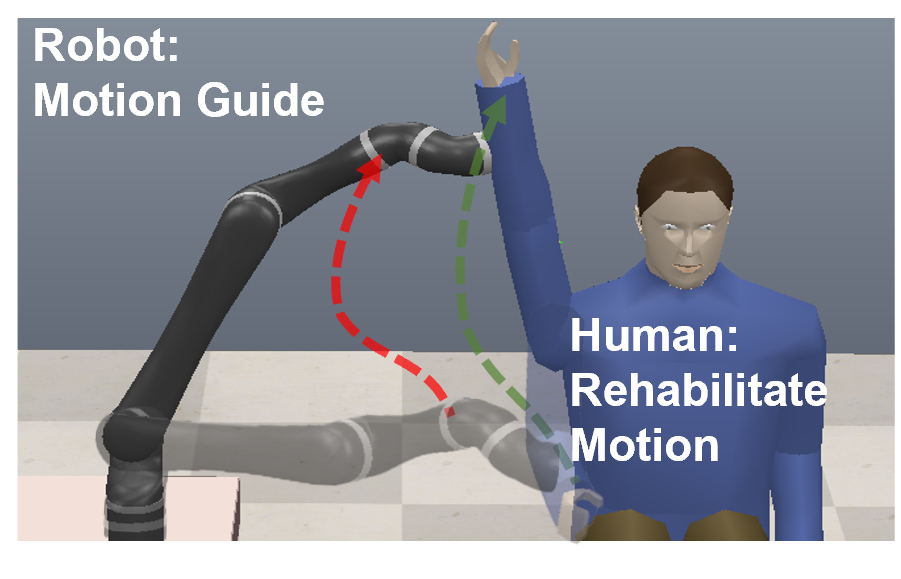
However, training a professional and safe rehabilitation robot is challenging due to clinical data scarcity. Besides treatment-relevant information (e.g., stroke types and motor impairments), clinical rehabilitation data also includes irrelevant but private information (e.g., patients’ identity, physiological characteristics, and other illnesses) [6]. Restrained by concerns of leaking patient information, clinical data cannot be accessed across hospitals; small-amount local data inadequately train a rehabilitation robot, further undermining its performance and safety and impeding widespread implementations of robotic rehabilitation [7]. Besides, patients vary in physical characteristics and motor impairments, adding challenges for robots to provide customized rehabilitation [8].
Therefore, to address the data scarcity issue, in this research, a novel joint training method – Federated Joint Learning (FJL) was developed to collaboratively train robots crossing hospitals. Particularly, our work in this paper mainly has three contributions:
-
•
A federated joint learning network was developed to network robots crossing hospitals and enable them to mutually learn rehabilitation skills from each other without directly accessing original patient data. Fig. 1 illustrates the simulation environment settings.
-
•
A LSTM-Transformer learning framework was developed to efficiently extract representative motion plans from complex spatiotemporal motions of patient joints with differences in body characteristics and motor impairment degree.
-
•
A novel relational loss was designed to refine the robot pose estimation result and improve the accuracy of the pose estimation model.
No comments:
Post a Comment