http://journal.frontiersin.org/article/10.3389/fncom.2016.00084/full?
- 1Grenoble Institut des Neurosciences, Université Grenoble Alpes, Grenoble, France
- 2Institut National de la Santé et de la Recherche Médicale, U1216, Grenoble, France
- 3GIPSA-Lab, Université Grenoble Alpes, Grenoble, France
- 4GIPSA-Lab, Centre National de la Recherche Scientifique, Grenoble, France
- 5Centre Hospitalier Universitaire (CHU) de Grenoble, Grenoble, France
- 6Pole Recherche, Centre Hospitalier Universitaire (CHU) Grenoble, Grenoble, France
- 7IRMaGe, Institut National de la Santé et de la Recherche Médicale US17 Centre National de la Recherche Scientifique UMS 3552, Grenoble, France
1. Introduction
In numerous neurological conditions, the adult central
nervous system retains an impressive capacity to recover and adapt
following injury. Such so-called spontaneous recovery occurs after
spinal cord injury, traumatic brain injury, and stroke. Therefore, a
basic understanding of the mechanisms that underlie spontaneous recovery
of function is the initial step in the development of modulatory
therapies that may improve recovery rates and endpoints (Nudo, 2013).
In acute stroke, it has been shown that initial damage disrupts
communication in distributed brain networks. This initial
disorganization is followed by a dynamic reorganization at subacute and
chronic stage that may determine the level of post-stroke recovery (Carter et al., 2012). Not only disorganization in structural connectivity has been reported and related to outcome of patients (Moulton et al., 2015) but also functional reorganization in the motor network of both ipsilesional and contralesional hemispheres (Loubinoux et al., 2003; Jaillard et al., 2005; Gerloff et al., 2006; Favre et al., 2014) to compensate for the lesion itself and for remote effects (see Grefkes and Fink, 2014
for a review). The role of the contralesional hemisphere in the
recovery process after stroke is supported by several studies using task
fMRI paradigms (Gerloff et al., 2006; Lotze et al., 2006; Riecker et al., 2010; Rehme et al., 2011; Teki et al., 2013; Grefkes and Fink, 2014)
but it has not been studied before as an independent network (without
taking into account the interhemispheric connectivity) of the brain. It
is thus of clinical interest to study the reorganization of the
contralesional hemisphere in stroke patients by means of functional
connectivity fMRI at rest.
In the recent years, there has been a great amount of
work developing new investigation methods of the brain connectivity
based on fMRI. Among those, the graph theoretical approach seems
particularly useful in the context of pathology since it underlines the
role of key communicating regions (hubs) in the graph. Since there was
no graph metric aiming at capturing this type of reorganization after
brain damage, the Hub Disruption Index (κ) was introduced in Achard et al. (2012)
to capture it. κ index summarizes graph metric changes at the nodal
level in a single value. It is thus a global index capturing changes at
the nodal level. For a given graph metric, κ is computed as the slope of
the linear regression model between the mean nodal metric value of a
reference group and the differential nodal metric value between a given
subject (patient or control) and that reference (see Figure 1 for a graphical explanation). If the subject's nodal values are close to those of the reference group (Figure 1C), the κ will be close to 0. Contrary, if the subject's nodal values are different from those of the reference group (Figure 1D),
with reduced values in nodes with high metric values in the reference
group, the κ will be negative. Once the reference group is computed, the
κ can be calculated for each control and each patient individually and
statistical tests can be applied to compare the differences between
groups.
FIGURE 1
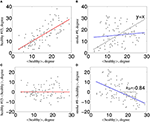
According to Bullmore and Sporns (2009),
hubs are crucial nodes for an efficient communication in the network
and are identified as nodes with high degree or high centrality values.
In this paper, we computed κ using metrics that directly relate to hubs:
node degree, betweenness centrality and global efficiency; and also in
metrics that explore the neighborhood of the node, such as, local
efficiency and clustering coefficient.
The aim of this paper is to quantify the impact of the
lesion on the brain network reorganization of the contralesional
hemisphere in severe stroke patients at subacute stage. For this
purpose, κ index is a perfect tool to assess such reorganization by
comparing nodal metrics between healthy volunteers and patients. In
order to translate the use of κ in clinical context, an essential
requirement to achieve meaningful results is to investigate the
reliability of this index. For this purpose, we used the intraclass
correlation coefficient (ICC), as it was previously assessed in several
studies working with brain graphs reliability in rs-fMRI (Schwarz and McGonigle, 2011; Wang et al., 2011; Braun et al., 2012; Guo et al., 2012; Liang et al., 2012; Cao et al., 2014).
This paper is divided into three parts: in the first
part, we assessed the reliability of κ, over different graph metrics, by
computing the ICC in a cohort of 100 healthy subjects using the
database from the Human Connectome Project (HCP)1. We calculated the ICCs and their p-values,
applying bootstrap and permutation techniques to check for the
influence of the number of subjects and of the number of edges (cost) in
brain graphs. We also explored whether there is a laterality effect by
testing the graphs of the intra-hemispheric connectivity from the left
and from the right hemispheres in healthy control subjects using the HCP
dataset. In the second part of the paper, we used the κ index to study
the reorganization that occurs in the contralesional hemisphere of 20
severe subacute stroke patients. Finally, in the third part, we tested
the robustness of the results obtained in this clinical study by
randomly choosing 20 subjects as “patients” and 20 subjects as
“controls” from the HCP database, computing the difference in κ between
them and replicating 1000 times this procedure.
No comments:
Post a Comment