The average MRI exam takes anywhere from 20-40 minutes and adding this on top of that will lead to many more neurons dying.
TIME IS BRAIN you know.
Maybe you want these much faster objective diagnosis options.
Hats off to Helmet of Hope - stroke diagnosis in 30 seconds; February 2017
Microwave Imaging for Brain Stroke Detection and Monitoring using High Performance Computing in 94 seconds March 2017
New Device Quickly Assesses Brain Bleeding in Head Injuries - 5-10 minutes April 2017
Ski-Mask Design AIR Coil Offers Whole-Brain Imaging Without Claustrophobia
Blood Biomarkers to Differentiate Ischemic and Hemorrhagic Strokes Might Allow Prehospital Thrombolysis
Approximate death rates; Your doctor will know exactly.
In each minute;
1.9 million neurons die
14 billion synapses die
12 km (7.5 miles) of myelinated fibers die
Brain ages 3.6 years each hour without treatment.
And all that damage is nothing compared to what you are going to lose by doing nothing to stop the 5 causes of the neuronal cascade of death in the first week. Your stroke doctors aren't even focusing on the real problem; the first week.
I lost 5.4 BILLION neurons in the first week.
The latest here:
Triage Brain MRIs with AI-Based System
Using a model trained on three data sets could lead to automated categorization of “likely normal” and “likely abnormal” brain MRI results.
MRI has grown in popularity as a tool to image the brain for various diseases or damage, leading to a heavy volume of scans for radiologists to interpret. Using an artificial intelligence (AI)-based system could help automate those interpretations to quickly identify the patients who need the most emergent care.
“MRI is increasingly used clinically for the detection and diagnosis of abnormalities. The resulting image overload presents an urgent need for improved radiologic workflow,” said a team of investigators from Massachusetts General Hospital (MGH) and Brigham and Women’s Hospital Center for Clinical Data Science. “Automatically identifying abnormal findings in medical images could improve the way worklists are prioritized, enabling improved patient care and accelerated patient discharge.”
The team, co-led by Romane Gauriau, Ph.D., former machine learning scientist from MGH, and Bernardo C. Bizzo, M.D., Ph.D., an MGH radiologist, published their findings Wednesday in Radiology: Artificial Intelligence. Their efforts resulted in a triaging system for brain MRIs that can detect multiple abnormalities from 3D volumetric imaging using a convolutional neural network and categorize the findings as “likely normal” and “likely abnormal.”
This is the first investigation to use a large, clinically relevant dataset and full-volume MRI data to identify overall brain abnormalities, they said.
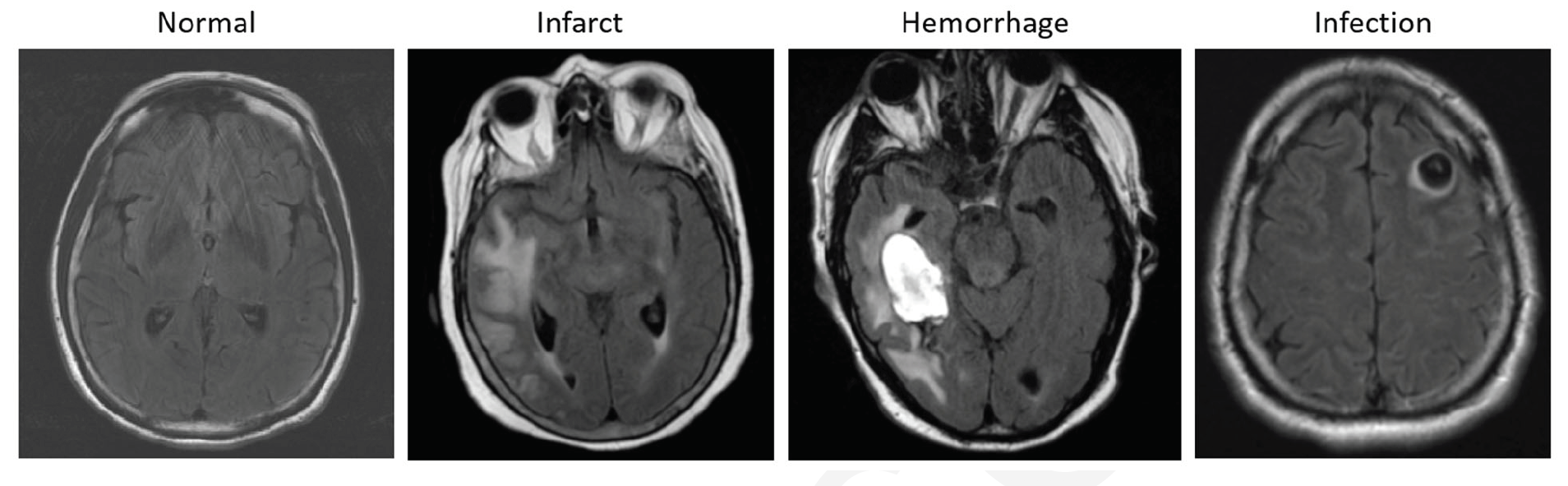
Examples of axial FLAIR sequences from studies within dataset A. From left
to right: a patient with a ‘likely normal’ brain; a patient presenting an intraparenchymal
hemorrhage within the right temporal lobe; a patient presenting an acute infarct of the
inferior division of the right middle cerebral artery; and a patient with known
neurocysticercosis presenting a rounded cystic lesion in the left middle frontal gyrus.
Credit: RSNA
For their retrospective study, the team used three large datasets that included more than 9,000 brain MRI scans culled from various institutions across two continents. They randomly split these datasets into training, validation, and test sets. All images were annotated by four radiologists who collated the studies into 10 categories: likely normal, almost normal, neoplasm, hemorrhage, infarct, infection or inflammatory disease, demyelination disease, vascular lesions, congenital, and other. In addition, FLAIR sequences were reviewed and categorized as “likely normal” and “likely abnormal.”
Based on their preliminary results, the team said, the model demonstrated relatively good performance in its ability to distinguish between “likely normal” and “likely abnormal” exams. Specifically, model A, trained on a dataset of axial FLAIR sequence exams conducted on adults and tested on a similar dataset that also included children, reached an F1-score of 0.72 and an area under the curve of 0.78 when compared with radiologic reports.
“Our experiments reveal reasonable performance for triaging purposes of a neural network for classification of likely normal or abnormal brain MRIs based on axial FLAIR sequences,” the team said. “Such a system could enable the creation of a ‘likely abnormal’ worklist which could be used to improve radiology workflow.”
With such a tool, they said, experienced radiologists and neuroradiologists could concentrate on the scans that were more urgent and time-consuming, and trainees and junior attendings could use it as a learning opportunity.
Their results also pointed to the generalizability of the model. They tested it on a validation set that was acquired during a different time period from a different institution than the data used the train the algorithm.
“The problem we are trying to tackle is very, very complex because there are a huge variety of abnormalities on MRI,” Gauriau said. “We showed that this model is promising enough to start evaluating if it can be used in a clinical environment.”
Like similar models that have been used to pinpoint abnormalities with CT scans and chest X-rays, this model has the potential to significantly improve turnaround time, as well as identifying incidental findings in outpatient settings.
“Say you fell and hit your head, then went to the hospital and they ordered a brain MRI,” she said. “This algorithm could detect if you have brain injury from the fall, but it may also detect an unexpected finding, such as a brain tumor. Having the ability could really help improve patient care.”
These findings point to the need for continued work, the team said. The next steps involve evaluating the model’s clinical utility and potential value for radiologist. Ultimately, they said, they wanted to be able further develop the model to provide the binary outputs “likely normal” and “likely abnormal.”
No comments:
Post a Comment