How are your doctor and hospital getting this research done in stroke subjects? Doing nothing? Then you don't have a functioning stroke hospital. Not sure what it is but it has nothing to do with stroke. RUN AWAY!
Accelerometers and motion sensors have been
written about forever. If your therapists don't use them, they have
nothing objective to base their therapy upon or recognize gains. That
is a fireable offense for the stroke department head and the stroke
hospital president.
-
accelerometers
(27 posts to June 2013)
-
accelerometry
(4 posts to February 2012)
-
motion sensors
(11 posts to August 2012)
Method for Estimating Temporal Gait Parameters Concerning Bilateral Lower Limbs of Healthy Subjects Using a Single In-Shoe Motion Sensor through a Gait Event Detection Approach
Biometrics Research Labs, NEC Corporation, Abiko 1131, Japan
*
Author to whom correspondence should be addressed.
Academic Editor: Wan-Young Chung
Sensors 2022, 22(1), 351; https://doi.org/10.3390/s22010351
Received: 1 December 2021
/
Revised: 22 December 2021
/
Accepted: 1 January 2022
/
Published: 4 January 2022
(This article belongs to the Special Issue Recent Advance and Application of Wearable Inertial Sensors in Motion Analysis)
To expand the potential use of in-shoe motion sensors (IMSs) in daily
healthcare or activity monitoring applications for healthy subjects, we
propose a real-time temporal estimation method for gait parameters
concerning bilateral lower limbs (GPBLLs) that uses a single IMS and is
based on a gait event detection approach. To validate the established
methods, data from 26 participants recorded by an IMS and a reference 3D
motion analysis system were compared. The agreement between the
proposed method and the reference system was evaluated by the intraclass
correlation coefficient (ICC). The results showed that, by averaging
over five continuous effective strides, all time parameters achieved
precisions of no more than 30 ms and agreement at the “excellent” level,
and the symmetry indexes of the stride time and stance phase time
achieved precisions of 1.0% and 3.0%, respectively, and agreement at the
“good” level. These results suggest our method is effective and shows
promise for wide use in many daily healthcare or activity monitoring
applications for healthy subjects.
1. Introduction
As
a result of the rapid development of wearable device technologies,
wearable smart motion sensors have been used in various healthcare
applications based on daily gait analysis, with all data processing
performed automatically on an edge device [1].
Recently, a new type of wearable smart motion sensor for daily gait
analysis, called an “in-shoe motion sensor” (IMS), was developed. The
IMS can be placed in various kinds of shoes or insoles, and is less
inconvenient to wear [2,3].
Thus, it shows promise for various healthcare and activity monitoring
applications, with the aim of improving the habits of healthy subjects
involving daily walking, by assessing temporal gait parameters or
detecting gait signal features through artificial intelligent
technologies [4].
Common
temporal gait parameters such as walking velocity, stride length, foot
angle, gait variability, cadence, and foot clearance can currently be
obtained in real time by automatic calculation in the microprocessor of
an IMS [4,5].
In contrast, temporal gait parameters concerning bilateral lower limbs
(GPBLLs) play more important roles in healthcare or daily activity
monitoring applications, such as evaluation of walking ability [4], metabolic evaluation [6], daily fatigue monitoring [7], and alcohol use monitoring [8].
These parameters include the double support time (DST), which is
defined as the duration when both bilateral lower limbs touch the ground
in one gait cycle (GC), and the symmetry index of stride time (SIStr) and symmetry index of stance phase time (SISta)
between the two limbs. These GPBLLs are important because they capture
the interaction of the two feet and are essential predictors for
estimating certain deep gait parameters, such as gait asymmetry, lower
limb muscle strength, and muscle strength transition ability from one
limb to the other [5,6,9].
Furthermore, these deep gait parameters are significant predictors for
metabolic monitoring, fatigue assessment, walking ability, body
functions, and alcohol monitoring [5,6,7,9,10,11,12].
To expand the potential use of IMSs in healthcare or daily activity
monitoring applications for healthy subjects, we believe it is necessary
to conduct automatic measurement of these GPBLLs by an IMS.
To obtain the temporal GPBLLs, which are illustrated in Figure 1,
it is necessary for foot motion signals to interact well with each
other. The motions of both feet are commonly detected by placing two
independent sensors on them. Measurement then requires the phase
difference between the motions in the same GC to be correctly traced on
the same temporal axis. A common approach is to temporally synchronize
the sensors using external devices, i.e., via a hardware connection [13,14].
In a smart system, the IMSs are synchronized with each other by
timestamps that originate from a universal time controller in the
system, e.g., a smartphone. This may make the system more complicated
and subject to the uncertainty in the sensor network, clock drift, and
packet delays in the communication protocol [15,16].
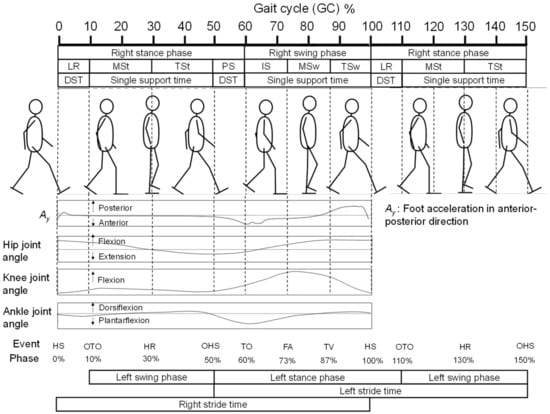
Figure 1.
Illustration of the definitions of a GC, gait phases, gait events, and
certain temporal gait parameters, and information on the foot
acceleration in the anterior–posterior direction, the knee joint angle,
and the ankle joint angle in one GC.
Accordingly, in this study, by combining
biomechanical knowledge with signal processing, we propose a new
approach to overcome these issues and provide a simpler method with
higher accuracy and precision for evaluation of temporal GPBLLs using
only a single sensor. Walking is a natural form of periodic movement.
Moreover, during walking, the motions of the two lower limbs
intrinsically interact well with each other, according to a
musculoskeletal model in which the phase difference between the two
limbs is locked by the connection of the pelvis. Thus, once the motion
of one foot is determined, the motion of the opposite foot can also be
known almost immediately [17].
A
GC is the time period or sequence of events or movements during
locomotion from when a foot contacts the ground, which is called a “heel
strike” (HS), to when that same foot again contacts the ground. The HS
is one of seven defined gait events, i.e., special motions during
walking. A GC can be partitioned into stance and swing phases, which
constitute 60% and 40% of the GC, respectively, as shown in Figure 1.
Furthermore, a GC can be divided into seven periods—loading response
(LR), mid-stance (MSt), terminal stance (TSt), pre-swing (PS), initial
swing (ISw), mid-swing (MSw), and terminal swing (TSw)—by the six gait
events in addition to HS: opposite toe off (OTO), heel rise (HR),
opposite heel strike (OHS), toe off (TO), feet adjacent (FA), and tibia
vertical (TV) [17].
Specifically, taking the right foot as an example, the OHS and OTO
events represent the respective moments when the left heel strikes the
ground and the left toe departs from the ground, which suggests that the
left foot’s exact temporal information for GPBLL estimation can be
obtained by detecting these two gait events from the right foot. Hence,
as long as the HS, TO, OHS, and OTO events of the right foot are
appropriately detected from foot motion signals, temporal GPBLLs such as
DST, SIStr, and SISta
can be captured well by a single IMS mounted on the right foot. In this
case, hardware connection of two sensors would no longer be necessary
to assess the interaction of two feet. We refer to this idea as the
“gait event detection approach”, which requires an IMS to have a
real-time gait event detection method.
In our previous study, we developed a rule-based real-time HS and TO detection method [18]. As shown in Figure 1,
we discovered that the foot acceleration signal in the
anterior–posterior direction exhibits a sharp valley next to the
maximum, which can be used for HS detection, while the first valley in a
W-shaped wave pattern appearing after a foot-flat state can be used for
TO detection. The missing piece of this approach is a method of
real-time OHS and OTO event detection for an IMS. Currently, many gait
event detection methods for wearable sensor systems have been proposed [19,20]. Previously, González et al. [21] proposed a method using a foot pressure sensor, and Liu et al. [22]
proposed a method based on fusion of data from multiple segments of the
lower limbs to detect OHS and OTO. However, these methods cannot be
used in an IMS system that only measures a single segment. For OHS and
OTO detection using foot-mounted inertial measurement units (IMUs),
Mariani et al. [23] developed hidden Markov models, and Kidziński et al. [24]
proposed a deep learning method. Such methods that construct
complicated models from training data require considerable processing
power and, thus, they are difficult to implement in a microprocessor
chip. In contrast, Rueterbories et al. [25]
developed a simpler, rule-based OHS and OTO detection method, which was
considered feasible for edge device processing. In their method, they
determine gait events through detection of peaks and valleys from three
types of low-pass-filtered composite signals, including a raw signal at
160 Hz and two moving-average raw signals with window lengths of 50 and
200; then, they analyze the relationships between detected feature
points. However, their method was sensitive to individual walking
velocity differences: when the deviation of the velocities from the
average increased, the precision of estimation decreased. To achieve
higher precision, improved OHS and OTO detection methods are thus
needed.
According to modern kinesiology studies
on walking, gait events correspond to the timings of muscle and joint
activity patterns transiting from one state to another [17,26].
Following these studies, we hypothesize that gait events should appear
as characteristic turning points, such as peaks, valleys, and
zero-crossing points, in the foot motion signal acquired by an IMS. In
the current study, we developed a rule-based method for OTO and OHS
detection and applied it to GPBLL measurements. To do so, we first used
biomechanical knowledge to analyze how the lower limbs move near the
moments of OHS and OTO [17],
and we then searched for possible feature points as candidates for OHS
and OTO detection based on the aforementioned hypothesis. Next, we
evaluated the accuracy and precision of our method through comparison
with the measurement results obtained by a 3D motion analysis system.
Given the similarity in motion between the left and right feet, if the
feasibility of our method is demonstrated by an IMS mounted on the right
foot, we assume that it should also be feasible for an IMS mounted on
the left foot.
More at link,
No comments:
Post a Comment