Absolutely no clue how this could possibly help in stroke recovery. Lots of pages to read on your own, unless you are under the mistaken impression that your doctor is responsible and will do something useful with this.
Experimental and Computational Study on Motor Control and Recovery After Stroke: Toward a Constructive Loop Between Experimental and Virtual Embodied Neuroscience
- 1Neuroscience Institute, National Research Council, Pisa, Italy
- 2European Laboratory for Non-Linear Spectroscopy, Sesto Fiorentino, Italy
- 3Department of Excellence in Robotics & AI, The BioRobotics Institute, Scuola Superiore Sant'Anna, Pontedera, Italy
- 4Aix-Marseille Université, Inserm, INS UMR_S 1106, Marseille, France
- 5Paris-Saclay University, Institute of Neuroscience, CNRS, Gif-sur-Yvette, France
- 6Department of Physics and Astronomy, University of Florence, Florence, Italy
- 7Biorobotics Laboratory, École Polytechnique Fédérale de Lausanne (EPFL), Lausanne, Switzerland
- 8Fortiss GmbH, Munich, Germany
- 9Bertarelli Foundation Chair in Translational NeuroEngineering, Institute of Bioengineering, Swiss Federal Institute of Technology (EPFL), Lausanne, Switzerland
- 10Chair of Robotics, Artificial Intelligence and Embedded Systems, Department of Informatics, Technical University of Munich, Munich, Germany
- 11Institute of Basic Medical Sciences, University of Oslo, Oslo, Norway
- 12Department of Biomedical Sciences, University of Padua, Padua, Italy
- 13Blue Brain Project (BBP), École Polytechnique Fédérale de Lausanne (EPFL), Geneva, Switzerland
1. Introduction
In nature, the activity of the brain of an individual
interacting with the environment is conditioned by the response of the
environment itself, in that the output of the brain is relevant only if
it has the ability to impact the future and hence the input the brain
receives. This “closed-loop” can be simulated in a virtual world, where
simulated experiments reproduce actions (output from the brain) that
have consequences (future input to the brain) (Zrenner et al., 2016). To the aim of reproducing in silico
the complexity of real experiments, different levels of modeling shall
be integrated. However, since modeling all components of an experiment
is very difficult, traditional approaches of computational neuroscience
reduce the experimental setups as much as possible. An “Embodied brain”
(or “task dynamics,” see Zrenner et al., 2016)
approach could overcome these limits by associating the modeled brain
activity with the generation of behavior within a virtual or real
environment, i.e., an entailment between an output of the brain and a
feedback signal into the brain (Reger et al., 2000; DeMarse et al., 2001; Tessadori et al., 2012).
The experimenter can interfere with the flow of information between the
neural system and environment on the one hand and the state and
transition dynamics of the environment on the other. Closing the loop
can be performed effectively by (i) validating the models on
experimental data, and (ii) designing new experiments based on the
hypotheses formulated by the simulations. On the example shown in Figure 1,
data on brain activity (be it, for instance, from electrophysiological
recordings or imaging) and on the environment (e.g., by means of
kinematic or dynamic measures) from the real experiment are used to feed
the models of the in silico representation of the experiment.
From a comparison of the real and model-based data, the features that
are most important to replicate the real experiment are identified, and
thus novel insights are generated (Figure 1).
To realize such a complex virtual system, many choices can be made, for
instance on the brain model or spinal cord model that best represent
the salient features of experimental measures to be replicated. The
ideal framework shall comprise a library of tools to choose from, to
reproduce a variety of experimental paradigms in the virtual
environment. By briefly introducing the state of the art in brain and
spinal cord modeling, we will discuss few classes of models to pick from
an ideal library.
FIGURE 1
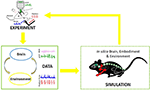
Lots more at link.
No comments:
Post a Comment